报告题目: Spontaneous Facial Activity Analysis by Modeling and Exploiting Comprehensive Interactions in Human Communication
报告时间:5月17日,星期四,上午10:00-12:00
报告地点:北五楼319
报告人:Yan Tong, associate professor, University of South Carolina
报告简介:
Driven by recent advances in human-centered computing, there is an increasing need for accurate and reliable characterization of the facial behavior displayed by users of a system. Recognizing spontaneous facial activity is challenged by subtle and complex facial deformation, frequent head movements, versatile temporal dynamics of facial action, especially when they are accompanied by speech. In spite of the progress on posed facial display and under controlled image acquisition, these challenges significantly impede spontaneous facial action recognition in practical applications. The major reason is that the information is extracted from a single source, i.e., the visual channel, as in the current practice. A strong motivation exists for finding a new scheme that can make the best use of all available sources of information such as audio and visual cues, as natural human communication does. Instead of solely improving visual observations, we seek to capture the global context of human perception of facial behavior in a probabilistic manner and to systematically combine the captured knowledge to achieve a robust and accurate understanding of facial activity.
In this talk, we will first present a novel approach that recognizes speech-related facial action units (AUs) exclusively from audio signals based on the fact that facial activities are highly correlated with voice during speech. Specifically, dynamic and physiological relationships between AUs and phonemes are modeled through a continuous time Bayesian network (CTBN). Then, we will present a novel audiovisual fusion framework, which employs a dynamic Bayesian network (DBN) to explicitly model the semantic and dynamic physiological relationships between AUs and phonemes as well as measurement uncertainty. Experiments on a pilot audiovisual dataset have demonstrated that the proposed methods yield significant improvement in recognizing speech-related AUs compared to the state-of-the-art visual-based methods. Drastic improvement has been achieved for those AUs, which are activated at low intensities or “invisible” in the visual channel. Furthermore, the proposed methods yield more impressive recognition performance on the challenging subset, where the visual-based approaches suffer significantly.
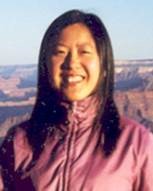
报告人简介:
Yan Tong received her BS degree in Testing Technology & Instrumentation from Zhejiang University in 1997, and a Ph.D. degree in Electrical Engineering from Rensselaer Polytechnic Institute, Troy, New York, in 2007. She is currently an associate professor in the Department of Computer Science and Engineering, University of South Carolina, Columbia, SC, USA. From 2008 to 2010, she was a research scientist in the Visualization and Computer Vision Lab of GE Global Research, Niskayuna, NY. Her research interests include computer vision, machine learning, and human computer interaction. She was a Program Co-Chair of the 12th IEEE Conference on Automatic Face and Gesture Recognition (FG 2017) and served as a conference organizer, an area chair, and a program committee member for a number of premier international conferences. She has received several prestigious awards such as USC Breakthrough Star in 2014 and NSF CAREER award in 2012.