报告题目:Constrained Deep Networks: Models and Optimization
报告时间:2019年6月27日,星期四,下午2:30-5:30
报告地点:数学楼2-3会议室
报告人:Ismail Ben Ayed 副教授,魁北克大学
报告摘要:
Embedding constraints on the outputs of deep networks has wide applicability in learning, vision and medical imaging. For instance, in weakly supervised learning, constraints can mitigate the lack of full and laborious annotations in dense prediction tasks, e.g., semantic segmentation. Also, adversarial robustness, which currently attracts substantial interest in the field, amounts to imposing constraints on network outputs. In this long talk, I will discuss some recent developments in those directions. A first part of the talk focuses on how to enforce various types of constraints and priors on weakly supervised convolutional neural networks (CNNs), which can leverage unlabeled data, guiding training with domain-specific knowledge. I will discuss several key technical aspects in the context of CNNs with partial labels, including constrained optimization, conditional random fields (CRF) regularization and computational tractability. In the second part, I will discuss stat-of-the-art models for adversarial robustness. In both parts, I will emphasize how more attention should be paid to optimization methods, going beyond standard gradient descent. The talk includes various illustrations, applications and experimental results.
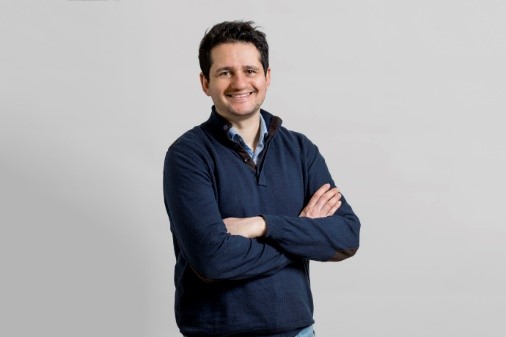
报告人简介:
Ismail Ben Ayed is currently Associate Professor at ETS Montreal, University of Quebec, where he holds a research chair on Artificial Intelligence in Medical Imaging. His interests are in computer vision, optimization, machine learning and medical imaging. Ismail authored over 90 fully peer-reviewed papers, mostly published in the top venues of the field, along with 2 books and 7 patents. In the last 5 years, he gave over 20 invited talks, 3 tutorials at flagship conferences (MICCAI’14, ISBI’16 and MICCAI’19) and 1 keynote at IEEE SIVA’18 conference. Recently, his research has been covered in several visible media outlets, such as Radio Canada (CBC), Quebec Science Magazine and Canal du Savoir. His team received several recent distinctions, such as MIDL’18 CIFAR student award, several top-ranking positions in internationally visible contests (e.g., NeurIPS’18 adversarial vision challenge and MICCAI’17 iSeg Challenge), Medical Physics Editor’s choice, highly competitive FRQNT fellowships, and 6 oral presentations at prestigious conferences such as CVPR/ECCV/NeurIPS (3% acceptance rate), among other recognitions. Ismail served as Program Committee for MICCAI’15, MICCAI’17 and MICCAI’19, Program Chair for IEEE IPTA’17, and will serve as Program Chair for MIDL’20. Also, he serves regularly as reviewer for the main publications of the field, and received the outstanding reviewer award for CVPR’15.
References
[1] Marin et al., Beyond gradient descent for regularized segmentation losses, CVPR’19
[2] Rony et al., Decoupling Direction and Norm for Efficient Gradient-Based L2 Adversarial Attacks and Defenses, CVPR’19 (oral presentation)
[3] Kervadec et al., Constrained-CNN losses for weakly supervised segmentation, Medical Image Analysis, 2019
[4] Tang et al., On regularized losses for weakly supervised CNN segmentation, ECCV’18
[5] Kervadec et al., Log-barrier constrained CNNs, ArXiv 2019